In 2023, Intech hosted a series of webinars on the role that data quality plays in driving effective and reliable artificial intelligence tools. The following article is the first in a series that will explore the themes that emerged from those webinars, as presented by our esteemed panelists who addressed the deep questions around the issues raised by the increasing prevalence of AI.
By TOBY WALSH (Laureate Fellow and Scientia Professor of Artificial Intelligence at the University of New South Wales and CSIRO Data61)
I’ve been working in the field of artificial intelligence for about 40 years now, and for most of that time, ‘AI’ was seen as something of an esoteric pursuit to which no-one really paid much attention.
But everything changed almost exactly a year ago to the day when OpenAI released ChatGPT, which captured people’s imaginations across the world.
Why was that? For someone working in the field, it was a bit of a surprise, because there’s already a lot of AI that’s ‘escaped the laboratory’, so to speak, and can now be found in many businesses.
A recent study estimated that some sort of AI touches your life 20 times a day. From every time you’re getting directions from your car’s GPS to every time you’re speaking to Siri or getting a film recommendation from Netflix, there’s some AI fueling that.
But the way ChatGPT has captured so many people’s imaginations shows that AI is starting to take on a more significant role in many aspects of our lives – and especially in business.
However, when we talk about AI today, many people can be misled to only think about AI as ChatGPT, or apps – or more broadly, generative AI – not realising that this is only a small piece of the much bigger AI puzzle.
Just like our own individual intelligence has many different dimensions to it, Artificial Intelligence also has many different dimensions.
And it’s not just the generative AI models, which are the flavour of the month. There are lots of different pieces to this AI jigsaw that fit together, broadly speaking. These include:
- Predictive AI (ie. machine learning) which is about making predictions based upon data.
- Natural Language Processing (NLP) – So much of our intelligence is bound up with our ability to use language, so NLP is a significant part of AI pursuits today.
- Data Mining – This is about looking at large datasets and finding patterns in the data, including and incorporating the speech recognition tools previously mentioned.
Perhaps what’s most important about this big AI picture, is the fact that there are still some missing pieces in the puzzle.
Putting the AI pieces together
Machines are still very limited in their capabilities, and we’re still working on building those up. Before we complete the AI puzzle, we will need to get machines to match humans in all their capabilities, including their reasoning capabilities. And, as just one example, tools like ChatGPT are very limited in this regard.
So why has AI captured our imagination now and not 30 years ago, after I’d been working in the field for 10 years? Why in 2023 is AI starting to capture so much attention and being so useful in so many practical applications? The answer is a story of exponentials.
You may hear a lot about how we live in “exponential times”, which is a bit of guff, really. Still, there are a couple of very important exponentials that have powered the ways that AI has started arriving in our lives. One is very well known by its name, Moore’s Law, which states that computing power is doubling every two years (which today is more like every 18 months.)
This has been going on now for more than 50 years and has brought some significant advances. Things I dreamt about doing just 10 years ago are now possible because we’ve got computers that are hundreds, in some cases, even thousands of times faster than they were, and millions of times faster than they were 20 or 30 years ago. We now have the raw brute force to be able to do these things.
However, there’s an equally important exponential that is very relevant to this discussion, and that’s the amount of data available to AI tools, which has also been doubling roughly every two years. Data is now measured in zettabytes, and there’s no physical reason why it should be the same measure as computing power. It’s a pure coincidence, as far as I can tell.
But it has been doubling. We’re connecting everything to the internet, we’re interconnecting all our devices ourselves and building the Internet of Things. There are also lots of businesses that are collecting much more data on their operations, their customers, and their markets – all of which are incredibly important for making decisions.
Indeed, one of the more valuable things that businesses are collecting now is data. And that growth in the amount of data we can access has been driving the AI revolution, because a lot of it comes back to machine learning – that is, getting machines to learn from data on how to do things.
The fact is, we now have such plentiful supplies of useful data that is really has made a significant difference to the success of Artificial Intelligence – and the doubling of data has been fundamental to that.
The importance of data quality
For those like me working in the AI field, the quality of available data became obvious for a very famous incident that took place 1999 during the upset in Eastern Europe, when the US accidentally bombed the Chinese Embassy in Belgrade. This turned out to be a data problem.
Because the US had some old data, they hadn’t noted that the Chinese Embassy had moved. This caused significant harm, sadly, with several people killed in the incident, which created a major rift in the relationship between the United States and China.
Following this however, there was a significant push from US funding agencies to use AI to try and improve the quality of the data that AI being trained upon. I saw it in my own work with some very large multinationals that were looking at optimising their supply chains.
We took their data on where their customers were and where their depots were, and when plotted on a map, we discovered there were depots in the middle of Sydney Harbour. Clearly, trying to pick up from depots in the middle of Sydney Harbour was going to be quite problematic! So, a significant part of that AI project became cleaning up the data so we could then throw the AI algorithms at it.
Ultimately, using data for AI comes down to the adage that’s been true for computer science since the very beginning – ‘Garbage in, garbage out’. That is, the quality of the solutions you can get from AI are entirely dependent upon the quality of the data that you’re providing to the algorithms.
As we’ve already discussed, that can be many things wrong with the data. What’s often called the ‘bitter lesson from AI’ is that you can spend a lot of time trying to get more sophisticated, more complex, smarter algorithms – or you can spend time trying to get better quality data.
It almost always turns out that the better investment of effort is in getting better quality data, rather than trying to get more sophisticated algorithms. If you get enough quality data, then even the simplest possible algorithms you can put data through tend to come up with good answers, as opposed to bad.
So, while plenty can be wrong with your data, the good news is that AI is not only part of the problem – ie. the reason for you wanting to clean up your data – it’s also partially the solution, and there are lots of AI tools that can help you deal with these issues.
Examples of issues that can arise in your dataset include missing values, duplicate data, erroneous data, and irrelevant data. These are things that you may have to address in your data to actually get it into a form that you can then throw algorithms at.
Every AI project begins with me typically asking a question of the people with whom we are collaborating, which is, ‘Tell me about your data’.
Once we understand what we are dealing with, we can then spend 80% of our time cleaning up the data – so that we then spend the right final 20% of the project applying AI methods for getting answers out.
It could not be clearer. Data quality is central to the success of making future progress in artificial intelligence.
Toby Walsh
Laureate Fellow and Scientia Professor of Artificial Intelligence at the University of New South Wales and CSIRO Data61
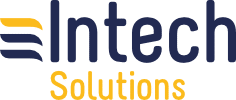
Intech provides data solutions that lay a secure foundation for robust, cost-effective and timely business transformation. Intech’s products have been successfully deployed to thousands of users, across hundreds of sites. See intechsolutions.com.au